Data Blending
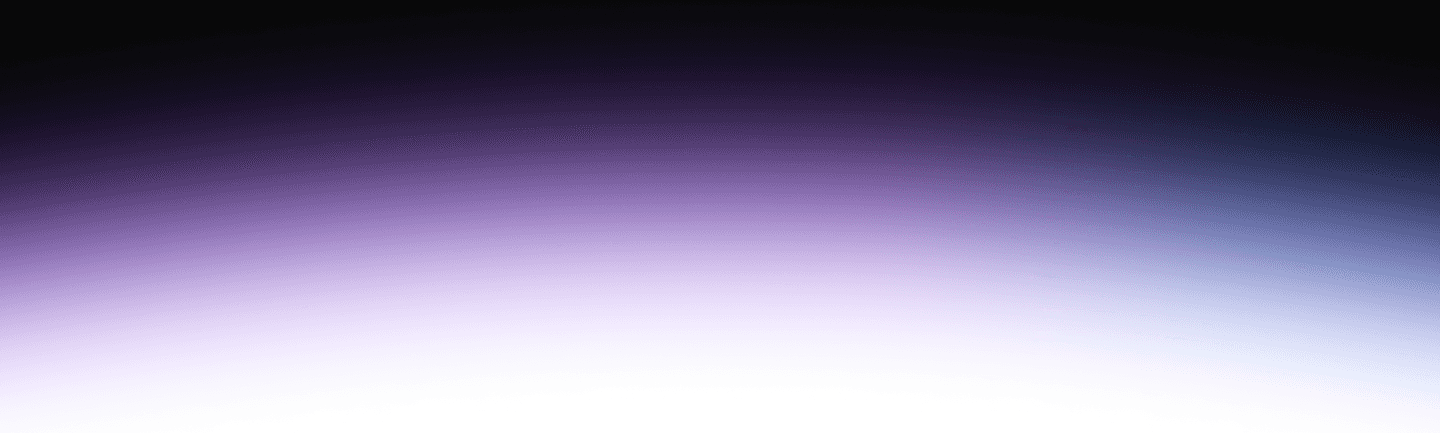
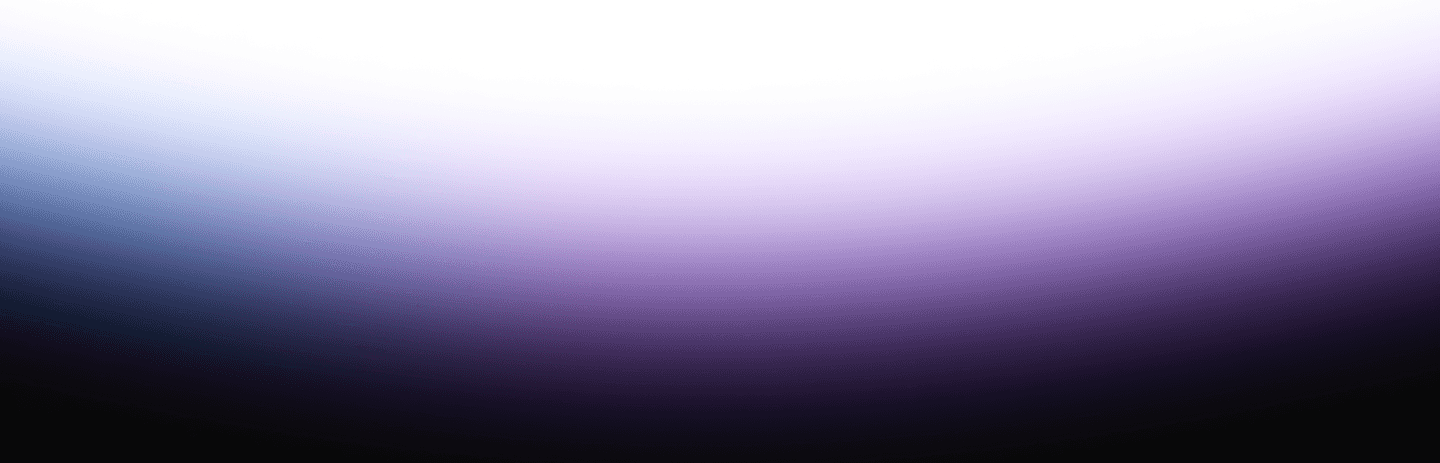
3 reasons to blend data
Blending’s accessibility and speed is an increasingly valuable tool for data-driven enterprises. Business leaders need the insights data offers to make rapid decisions in dynamic market conditions.
Expanding data complexity far exceeds the capabilities of Microsoft Excel spreadsheets and other traditional decision-support tools. Data teams have the skills and infrastructure to process complex data. However, their time is over-subscribed, and pipeline development takes too long.
Blending brings big data analytics closer to where decisions happen. Here are a few reasons to blend data:
1. A method of data discovery and data exploration
Data science projects use data the company may or may not have to surface questions yet to be asked and reveal insights that shape long-term strategy.
One role for blending is supporting ad hoc reporting to answer business questions. Using the data the company has right now, analysts can inform business leaders’ tactical decisions.
Applying blending to discovery and exploration lets analysts create fresh combinations of data from SQL databases and other sources regardless of organizational or regional boundaries.
2. Richer dataset
Individual data sources can only answer a limited set of questions. One reason companies build data warehouses is to give analysts a richer data pool. However, data warehouses are limited in the amount and variety of data they can store.
Blending gives analysts access to a broader range of data. They can combine different types of data from many more sources. A warehouse may be the analyst’s primary data source. Secondary data sources, such as customer relationship management systems or demographic databases, enrich the blended data set with context to support more nuanced analysis.
3. Cross-functional collaboration
Blending’s boundary-crossing access and ease of use foster collaboration in data-driven business cultures. Stakeholders from manufacturing, sales, finance, and other departments must have a shared consensus about the data used to plan and execute a project. Blending tools pull data directly from sources in each domain rather than from an unfamiliar intermediate source. As a result, there’s no project-delaying debate about how “real” the numbers are.
Impromptu collaboration is much easier when cross-functional teams can use blending tools without requesting ETL pipeline development. Business analysts can help with the initial setup or dashboard creation. At the same time, any team member has the power to run numbers or generate graphs.
How does data blending work?
Analysts use blending to support particular business needs, so understanding decision-makers’ requirements is a prerequisite to anything else. They need to know the business context of the questions and the kind of information the business needs to address the task at hand. With clearly defined objectives, analysts can begin the five-step data blending process.
Prepping the Data – Analysts use these objectives to identify relevant data sources and evaluate data sets within each source. A key factor is whether the data sets share at least one common dimension that connects sets from each source and allows blending to work. During discovery, analysts also map source-to-source variations in schema, types, formats, metadata, and other properties for use later in the blending process.
Merging the Data – Analysts next structure the final data set, using the project requirements to choose which data to include from each source. This prioritization optimizes the final data set for storage and performance. In addition, limiting the data set’s scope makes it easier for downstream users. With a structure in place, analysts extract and merge data from each source, aligning everything along the shared dimension.
Cleansing the Data – This merged data set won’t be fit for use immediately. Analysts must address missing, duplicate, or outlying data. Cleansing enhances the final product’s data quality as well as the quality of any insights derived from the data.
Validating the Data – Validation is a higher-level quality check. Analysts identify, investigate, and address cases of unmatched records to ensure completeness. They also check the consistency of formats and other data properties. Once the data set is ready, it loads into the final destination for analysis.
Visualizing and Analyzing the Data – Whether loaded into a database or a data warehouse, the blended data set becomes accessible to business intelligence apps and data visualization tools that support the project’s business needs.
Data architectures for data blending
Combining multiple sources into a single data set is not as straightforward as loading everything into a spreadsheet. The variety and volume of data used in modern decision-making require more advanced data architectures.
Data warehouses
Under certain conditions, a data warehouse can be a centralized repository for the blended data set. Data warehouses cannot handle data like images or text files, so they only accept structured data. In addition, data warehouses combine compute and storage capabilities, which requires worst-case investments in both to guarantee availability and performance.
Data lakes
Data lakes are centralized repositories for structured and unstructured data. Ingestion pipelines perform minimal processing to preserve the data in its raw format, making data lakes a better fit for the blending process. Furthermore, data lakes are storage solutions that separate analytics software access. Separating storage from compute allows more efficient resource allocation based on actual demand.
Data blending use cases examples by industry
Any organization can use data blending to bring big data analysis closer to business decision-making. Here are six industry use cases that demonstrate data blending benefits.
Supply Chain Management – Blending data from third-party suppliers and transportation providers, as well as internal distribution centers and logistics systems, optimizes inventories and expenses.
Healthcare – Hospital executives combine data from laboratories, health information systems, and other sources to better understand how treatments affect patient outcomes.
Energy and Utilities – During extreme weather events, power companies blend data from their own plants and a network of third-party gas, solar, and wind providers to balance loads and minimize disruptions.
Financial Services – Financial institutions rely on compliance analysts to quickly blend data from multiple sources when investigating potential violations of money laundering or terrorist financing.
Retail and E-commerce – Dynamic omnichannel marketing strategies require iteration and agility, which only blending’s quick access to sales data from stores, websites, apps, and other sources provides
Manufacturing – To optimize production, manufacturers will run experiments that require ad hoc blending of data from suppliers, sensors, laboratories, and many other sources.
Data blending tools
Blending’s biggest advantage is how it lets data users with different levels of technical sophistication analyze complex data. At one end of the spectrum, people view dashboards or pull data into an Excel worksheet. At the other end, experienced data analysts can use powerful analytics tools such as:
Tableau – Data blending in Tableau is a powerful way to present complex information visually and create compelling data stories.
Alteryx – Analysts use Alteryx drag-and-drop building blocks to create no-code automations for analyzing blended data.
Power BI – Integrations with Microsoft’s enterprise ecosystem let Power BI users seamlessly analyze, visualize, and present insights from blended data.
Challenges with data blending
The limitations of data blending can introduce friction that prevents business leaders from getting the answers they need quickly.
Managing complexity
Unlike the monolithic structures of textbooks, enterprise data architectures scatter sources across multiple systems, domains, and geographies.
Data architecture complexity extends the early stages of data blending, negating blending’s speed and accessibility advantages. Finding the right data requires more time from analysts comfortable navigating the company’s data labyrinth.
In addition, project complexity impacts scalability and performance when blending must create enormous data sets from thousands of disparate sources.
Ensuring consistency
Complexity also affects the cleansing and validation stages. Without centrally coordinated data governance, data sources will vary in quality, provenance, structure, and format. These inconsistencies are inconvenient when blending a few sources. However, they become a scalability challenge when turning data from thousands of independent sources into accurate, high-quality blended data sources.
Maintaining security
Blending data while complying with governance rules adds another obstacle to insight generation. Regional privacy regulations limit access to personally identifiable information and may prevent the granularity a project requires. Compliance with security standards may also affect what data a blending project may access.
Data blending with Starburst
Starburst’s modern data lake analytics platform provides the seamless integration of disparate data sources and democratized access needed to support ad hoc reporting and other business needs through data blending. Starburst Galaxy creates a virtual access layer that leaves data at the source, providing a single point of access to data stored across the enterprise.
Analysts can use familiar SQL tools to discover, prepare, merge, clean, and validate blended data. There is no need for dedicated data warehouses or lakes since Starburst’s performant query engine generates near real-time results. Analyzing and visualizing blended data becomes much simpler, giving business leaders the answers they need to make informed decisions.